Linear panel data models
Random effects model are statistical models in the analysis of panel data are used in which several individuals are observed over several time periods. Panel data models use this panel structure and allow unobserved heterogeneity of individuals to be taken into account. The two most important linear panel data models are the panel data model with fixed effects ( English fixed effects model ) and the panel data model with random effects ( English random effects model ). The two models differ in the assumptions made on the model's error terms and allow different estimators to be derived . Areas of application of linear panel data models can be found primarily in empirical social research .
Basics
When evaluating statistical data, statements about the underlying distribution of characteristics in a population should be determined from a finite amount of data . One tries to determine the unknown parameters of this population with the help of estimators . A typical application is to estimate the effect of one variable on another variable (see also regression analysis ). An example of this would be the relevant question in labor economics about the effect of education ( ) on a person's income ( ).
An estimator is a random variable , which leads to a lack of clarity in the determined parameters (see also distribution function and variance ). Therefore, even in the ideal case, the “ true value ” of the unknown parameter is not achieved, but only approximate values.
The ability to reach the true value at least in the expected value ( faithfulness to expectations ) or to converge against it for large samples ( consistency ), as well as the variance of the estimator around the true value are therefore important properties of an estimator. The least squares method is a widely used method to construct estimators that under the Markov assumptions Gaussian consistently and efficiently are. If, however, relevant quantities are not included in the regression, endogeneity , heteroscedasticity and autocorrelation can arise, as a result of which the least-squares estimation loses its desirable properties and becomes inefficient or even inconsistent. Using panel data and panel data models, estimators can be derived that solve these problems.
A typical equation of a linear panel data model for a panel with individuals and time periods has the form
- .
It shows the characteristics of the declared / dependent variable for the individual and time period . Is a vector that contains the characteristics of the explanatory / independent variables . An example could be a person's income in the year . Variables in the vector would then be those factors that have an influence on a person's income, such as age, work experience, whether a person is unemployed or not, gender, nationality or the number of training seminars attended. The variables combined in the vector can all be observed and are available in the data set. In addition to these variables, however, there are other factors that cannot be observed or can only be observed with great difficulty and are therefore not available in the data set. These factors are represented by the terms and . represents a collective term for all those unobserved variables that differ over time and people, for example the health of a person in the year . stands for the unobserved variables that differ between people, but are constant over time for a given person. Examples of this would be a person's fundamental values or their intelligence / ability. The terms are known as “unobserved heterogeneity”, “ latent variable ” or “individual heterogeneity” , among other things .
As an alternative to the above notation, a matrix notation is often used in which the individual equations are quasi “superimposed”. This then gives the model
- .
There is a vector with the characteristics of the explained variable, a matrix with the characteristics of the explanatory variables. is a vector with the coefficients of the explanatory variables, and and are vectors with the error terms.
The two main linear panel data models are the fixed effects model and the random effects model . The main difference between these two models is the assumption made about the correlation between individual heterogeneity and the explanatory variables observed.
example
An example of the application of models with random effects and models with fixed effects and their estimators can be found in the above question about the influence of education on a person's income. As mentioned above, a person's annual income would be the declared variable; explanatory variables would be education (measured in years or in completed classes / courses), the effect of which is to be measured. In addition, all variables that are correlated with income and education would have to be included in the regression. Examples would be the age, the professional experience or the education of the parents. In addition, it is possible that other relevant factors (for example, intelligence, health or a person's values) are not recorded - so there will be individual heterogeneity. One possible equation to estimate would be
where represents a vector with additional control variables such as age, experience and the like. The variable includes not only the education completed before starting work, but also qualifications acquired later. will absorb all effects that are constant in an individual over time, but cannot be included in the regression as control variables, for example because they cannot be directly observed. As mentioned earlier, the intelligence of the observed individuals is an example of this. This will likely have an impact on an individual's earnings and will also be correlated with education. However, intelligence is difficult to measure and therefore difficult to include as a control variable in the regression. The same applies to other unobserved but relevant variables that together form “individual heterogeneity”. The correlation between this heterogeneity and the explanatory variables is the central difference between the model with random and that with fixed effects. If there is no such correlation, the model with random effects is used. The fixed effects model is used when individual heterogeneity is correlated with explanatory variables.
Model with random effects
Basics
The model with random effects (sometimes also called a model with a random intercept for delimitation ) makes the assumption that the unobserved heterogeneity is orthogonal to the explanatory variables, i.e. does not correlate with the explanatory variables:
In addition, strict exogeneity of the error term must also be assumed:
- .
Under these assumptions, individual heterogeneity can be seen as another error term; H. the model to be estimated can be rewritten as
With
- .
Based on the above assumptions, then for .
The random effects model thus satisfies the requirement that the regression error term and the explanatory variables are uncorrelated. For this reason, ordinary least squares estimation would lead to consistent estimates for . Due to the individual heterogeneity, however, the model with random effects does not meet the assumption that the error terms are uncorrelated . Even if
and
Are constants and the idiosyncratic error terms are uncorrelated ( , ), there will be a correlation between the composite error terms of the same individual for different times:
For this reason, is variance-covariance matrix one - diagonal matrix be given by
- ,
where the individual diagonal elements are given by matrices
- .
The matrix is therefore not a diagonal matrix , but a block diagonal matrix . The special structure with only two parameters ( and ) is also known as the RE structure.
On the basis of this model, several estimators can be derived that are consistent and possibly also efficient.
Estimator in the model with random effects
Least Squares Estimation
As stated above, in the random effects model, the composite error term and the explanatory variables are uncorrelated, which is why the least squares method leads to consistent estimates. In connection with panel data, ordinary least squares estimation ( OLSE ) is also referred to as pooled least squares estimation ( pooled OLS ) because the panel data is pooled (over both groups), d. H. the time structure of the panel data is disregarded and the model is estimated on the basis of the pooled data with least squares estimation.
However, a matrix with the RE structure does not fulfill the assumption, which is central to the Gauss-Markow theorem , that the error terms are uncorrelated, which a diagonal variance-covariance matrix with a constant diagonal element requires. The least squares estimation is therefore not necessarily efficient in the model with random effects . In addition, the least squares estimated standard errors are incorrect because they ignore the correlation over time. For inference and hypothesis tests , the standard errors would have to be adjusted.
Random Effects Estimator

The “estimator for random effects” (“RE estimator”) provides a remedy at this point. Specifically, this is the estimated generalized KQ estimator , or GVKQ estimator for short, applied to the model with random effects . Suppose the variance-covariance matrix were known. Then the model could be transformed by multiplying it by on both sides :
If one now sets , then is the variance-covariance matrix of the error term in the model transformed in this way
- .
There
- applies, consequently applies .
If the variance-covariance matrix were known, the model could be transformed by it in such a way that the transformed model would have the identity matrix as the variance-covariance matrix. This identity matrix would satisfy the assumptions of Gauss-Markow's theorem , so the estimator would be efficient. This hypothetical model that is applicable not only to random effects model, but to all linear models with heteroskedasticity and autocorrelation can be as generalized least squares estimation , short VKQ ( English Generalized Least Squares , shortly GLS known). In the model with random effects, however, the exact variance-covariance matrix is unknown, so the VCQ estimation cannot be carried out. But instead, the so-called estimated generalized least squares estimation ( English Estimated Generalized Least Squares , in short: EGLS ) are applied, a two-stage procedure.
Here, the underlying model is first estimated with a least squares estimate, which, as explained above, leads to consistent estimates. On the basis of this least squares estimate and its residuals , consistent estimators and can then be calculated and an estimated variance-covariance matrix can be constructed with them . is then used to transform the underlying model:
- .
This transformed model is then estimated again using the least squares estimate, which results in the GVKQ or estimator for random effects:
- .
As a member of the GVKQ family, the estimator for random effects also has the same desirable properties as other GVKQ estimators: It is asymptotically equivalent to the VKQ estimator and therefore asymptotically efficient. For a simple implementation of the estimator for random effects, modern statistical programs can use routines that have already been programmed.
Between estimator
Another consistent estimator in the model with random effects is the so-called “between estimator”. A kind of cross-sectional structure is created by forming mean values:
- ,
where all mean values were calculated over time, for example . The between estimator is then calculated using a least squares estimate of the model expressed in mean values. It is consistent if and the composite error term are uncorrelated. In the model with random effects, this is due to the orthogonality assumption
the case and the between estimator are consequently consistent.
Potential problems
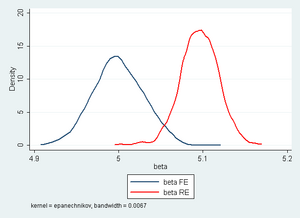
The central assumption of the random effects model is that the unobserved individual heterogeneity is not correlated with the other explanatory variables. If, however , the model with random effects is not applicable, the random effects, least squares and between estimators are inconsistent.
Fixed effects model
Basics
The model with fixed effects (also known as fixed effects model , FE model for short ) and the estimators based on it make it possible to consistently estimate the effects of the explanatory variables even if the individual, time-constant heterogeneity is correlated with the explanatory variables.
Estimator in the fixed effects model
Fixed Effects Estimator / Within Estimator
The basic idea of the estimator for fixed effects is to remove the individual heterogeneity from this by means of a suitable transformation of the estimation equation. On the one hand, the panel or multilevel structure of the data is used, on the other hand, the assumption that the individual heterogeneity is fixed , i.e. a constant specific to each individual.
The underlying model is again
- .
Furthermore, the assumption of strict exogeneity with regard to , i.e. H.
- .
In contrast to the model with random effects, however, can be. If so, so is
and ordinary least squares or RE estimation will not be consistent in this case.
One remedy is the so-called estimator for fixed effects (sometimes also called within estimator ). The idea here is to eliminate the individual-specific heterogeneity that is constant over time by subtracting the individual-specific average over the time periods from each observation. So the model to be estimated becomes
where (and analogously for the other variables) applies. In this case, the individual-specific heterogeneity (the “fixed effect”) falls out of the model. The estimator for fixed effects then results from an ordinary least squares estimation of the transformed model. The FE or Within estimator is consistent: Da , is in the transformed model , i. H. the error terms and their time averages are not correlated with the explanatory variables and their time averages. Assuming that the error terms for an observation unit have a constant variance over time and are not correlated with one another, the within estimator is also efficient.
It can also be shown that the Within estimator is asymptotically normally distributed . Assuming homoscedasticity and no autocorrelation of the error terms, the asymptotic variance of the estimator can be calculated as
It is the variance of the error term u . To estimate the variance, all that is then required is a consistent estimator of the error term variance. Such is given by
If the homoscedastic assumption is to be deviated from, the variance can also be estimated using a “robust” estimator. This is in the case of the within estimator
- .
On the basis of the estimated variance, hypothesis tests can then be carried out and confidence intervals calculated.
Instead of the described transformation of the model by subtracting the individual averages over time, other estimators can also be used. The so-called least-squares estimator using dummy variables or OLSDV estimator ( English OLSDV for ordinary least squares dummy variable ), for example, the explanatory variables of the model adds yet dummy variables for each observation unit added; then an ordinary least-squares estimation of this extended model is performed. With the help of the Frisch-Waugh-Lovell theorem it can be shown that the resulting estimators for the coefficients are identical to those of the estimator for fixed effects. In addition, the LSDV regression also gives estimates for the individual terms . However, these are only consistent if the number of time periods is large.
First difference estimator
Another possibility to address the problem of individual heterogeneity with the help of panel data methods is the formation of differences, which leads to the first difference estimator. The observation that preceded one period is subtracted from each observation:
- .
Since the individual heterogeneity is assumed to be constant over time, it falls out here, and the model in differences can be estimated by least squares estimation. If it is assumed that the error terms in the regression are homoscedastic and uncorrelated over time, the within estimator (= estimator for fixed effects) is more efficient than the first difference estimator. On the other hand, under the weaker assumption that the first differences in the error terms are uncorrelated over time, the first difference estimator is more efficient.
Potential problems
A common problem with using estimates in the fixed effects model is when the underlying data was collected with a measurement error. Incorrect data collection is a problem even in normal least-squares estimates based on cross-sectional data, which can lead to inconsistent estimates. The transformation on which the within estimator is based can further increase this error. An example of this is a study by the American economist Richard B. Freeman from 1984. At that time, fixed effects estimates were often used to estimate the causal effect of union membership on a worker's earnings. The underlying reasoning was that workers who join a union also differ in other, unobservable characteristics from workers who are not union members. Because of these assumed systematic differences, panel data and estimators for fixed effects were ideal. Freeman's results showed, however, that the results of the fixed effects are skewed downwards due to erroneous data collections, while ordinary least squares estimates based on cross-sectional data are skewed upwards; Both techniques do not allow a consistent estimate in this case, but the results of the fixed effects can be viewed as the lower limit and the least squares results as the upper limit for the underlying effect.
One possible remedy for problems due to incorrect data collection is to apply an instrument variable strategy . For example, if there are two measurements of a variable, one of them can be used as an instrument for the second measurement, which then allows a consistent estimate of the effect of the double measured variable.
Another problem is that the calculation based on deviations from the mean not only corrects the unobservable individual heterogeneity, but also removes part of the variation in the explanatory variables - both “good” and “bad” variation are produced from the model away. This becomes clearest with explanatory variables that are constant over time: These are completely removed from the estimation equation by the within estimator and the difference estimator. This is also a problem for the example of the regression of income on education mentioned at the beginning: The education acquired before working life is a constant from a later point of view, so the remaining variation in the model is primarily based on qualifications acquired later. The applicability of estimators for fixed effects to this model was therefore disputed as early as the 1980s. In a paper from 1981 Jerry Hausman and William E. Taylor showed a way how, under additional assumptions on the data, coefficients for variables constant over time can also be estimated in the fixed effects calculus.
Comparison of both models
The decision as to whether and which estimator of the model with random effects or the model with fixed effects should be used depends on the nature of the underlying model. If the underlying model has the fixed effects structure (i.e. a correlation between individual heterogeneity and explanatory variables), the within estimator is consistent and the estimator for random effects is inconsistent. If, on the other hand, there is an RE structure, then both the within estimator and the estimator for random effects are consistent, but the estimator for random effects is more efficient, i.e. it has a smaller variance and thus allows a more precise estimate. In order to decide which model is available, it is possible to carry out the Hausman specification test . The differences between the two estimators are compared; if these are large from a statistical point of view, this is viewed as an indication of the existence of a model with fixed effects.
Linear panel models also reach their limits when the explained variable in a time-delayed form is also an explanatory variable, for example as
In such a model, no consistent estimates are possible with the conventional estimators based on linear panel models. In such cases, dynamic panel data models must therefore be used. Estimation methods here are the Arellano-Bond estimator, which is close to the fixed effects calculus (after Manuel Arellano and Stephen Bond ) and the Bhargava-Sargan estimator, which is similar to the random effects calculus (after Alok Bhargava and John Denis Sargan ).
literature
- Joshua D. Angrist and Jörn-Steffen Pischke : Mostly Harmless Econometrics: An Empiricist's Companion , Princeton University Press, 2008
- Badi. H. Baltagi: Econometric Analysis of Panel Data . 5th edition. John Wiley & Sons, 2013.
- A. Colin Cameron and Pravin K. Trivedi: Microeconometrics- Methods and Applications , Cambridge University Press, 2005, ISBN 0521848059 , especially chapter 21
- Jeffrey M. Wooldridge: Econometric Analysis of Cross Section and Panel Data: Second Edition , Cambridge: MIT Press, 2002, especially Chapter 10
- STATA FAQ: What is the between estimator? . Retrieved October 5, 2011.
- STATA FAQ: Fixed, between, and random effects and xtreg . Retrieved October 5, 2011.
- Ludwig Fahrmeir , Thomas Kneib , Stefan Lang: Regression: Models, Methods and Applications. 2nd Edition. Springer Verlag, 2009, ISBN 978-3-642-01836-7 .
Remarks
- ↑ Ludwig Fahrmeir , Thomas Kneib , Stefan Lang: Regression: Models, Methods and Applications. , Springer Verlag 2009, p. 253
- ↑ Ludwig Fahrmeir , Thomas Kneib , Stefan Lang: Regression: Models, Methods and Applications. , Springer Verlag 2009, p. 253
- ↑ For an overview, see, among others, David Card : Estimating the Return to Schooling: Progress on Some Persistent Econometric Problems , Econometrica , 69.5, September 2001, pp. 1127–1160
- ^ Wooldridge, Econometric Analysis of Cross Section and Panel Data , 2002, p. 251
- ↑ It would also be conceivable to assume as the third unobserved term a term that is constant between people but changes over time , which stands for unobserved variables that change over time but affect all individuals equally, for example economic developments.
- ↑ See for example Joshua D. Angrist and Whitney K. Newey: Over-Identification Tests in Earnings Functions with Fixed Effects , Journal of Business and Economic Statistics 9.3, p. 321
- ↑ Cameron & Trivedi, Microeconometrics , 2005, p. 700
- ^ Wooldridge, Econometric Analysis of Cross Section and Panel Data , 2002, p. 257
- ^ Wooldridge, Econometric Analysis of Cross Section and Panel Data , 2002, p. 258
- ^ Wooldridge, Econometric Analysis of Cross Section and Panel Data , 2002, pp. 258f.
- ^ Wooldridge, Econometric Analysis of Cross Section and Panel Data , 2002, p. 259
- ↑ Cameron & Trivedi, Microeconometrics , 2005, p. 702
- ↑ Cameron & Trivedi, 2005, Microeconometrics , p. 703
- ↑ For further details, see Cameron & Trivedi, Microeconometrics , 2005, p. 82
- ↑ Ludwig von Auer : Econometrics. An introduction. Springer, ISBN 978-3-642-40209-8 , 6th, through. u. updated edition. 2013, p. 408.
- ↑ For the exact calculation see Wooldridge, Econometric Analysis of Cross Section and Panel Data , 2002, pp. 260f.
- ↑ Cameron & Trivedi, Microeconometrics , 2005, pp. 81f.
- ^ Wooldridge, Econometric Analysis of Cross Section and Panel Data , 2002, p. 260
- ↑ Cameron & Trivedi, Microeconometrics , 2005, p. 703
- ↑ Cameron & Trivedi, Microeconometrics , 2005, p. 726
- ↑ Cameron & Trivedi, Microeconometrics , 2005, p. 726
- ^ Wooldridge, Econometric Analysis of Cross Section and Panel Data , 2002, pp. 269f.
- ↑ Cameron & Trivedi, Microeconometrics , 2005, p. 727
- ↑ Cameron & Trivedi, Microeconometrics , 2005, pp. 732f.
- ^ Wooldridge, Econometric Analysis of Cross Section and Panel Data , 2002, pp. 279–281
- ↑ Angrist & Pischke, Mostly Harmless Econometrics , 2009, p. 225
- ^ Richard B. Freeman: Longitudinal Analyzes of the Effects of Trade Unions , Journal of Labor Economics , January 2.1, 1984, pp. 1-26
- ↑ Angrist & Pischke, Mostly Harmless Econometrics , 2009, p. 226f.
- ↑ For examples, see, for example, Orley Ashenfelter & Alan B. Krueger : Estimates of the Economic Returns to Schooling from a New Sample of Twins , American Economic Review , 84.5, 1994, pp. 1157–1173 or Andreas Ammermüller & Jörn-Steffen Pischke : Peer Effects in European Primary Schools: Evidence from the Progress in International Reading Literacy Study , Journal of Labor Economics, 27.3, 2009, pp. 315-348
- ↑ Angrist & Pischke, Mostly Harmless Econometrics , 2009, p. 226
- ^ Wooldridge, Econometric Analysis of Cross Section and Panel Data , 2002, p. 266
- ^ See, for example, Jerry A. Hausman and William E. Taylor, Panel Data and Unobservable Individual Effects , Econometrica, 49.6, 1981, pp. 1377f .; Angrist & Newey, Over-Identification Tests in Earnings Functions with Fixed Effects , Journal of Business and Economic Statistics 9.3, on the other hand, argue that post-school education for adult men in the USA still shows some variance and can therefore be viewed as time-variant.
- ↑ See Jerry A. Hausman and William E. Taylor, Panel Data and Unobservable Individual Effects , Econometrica 49.6, 1981, pp. 1377–1398
- ^ Wooldridge, Econometric Analysis of Cross Section and Panel Data , 2002, p. 288
- ↑ For the example of the Within estimator, see Cameron & Trivedi, Microeconometrics , 2005, p. 763f.