History of the calculus of probability
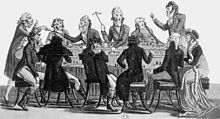

The history of probability theory or stochastics describes the development of a simultaneously ancient and modern branch of mathematics that deals with the mathematical analysis of experiments with uncertain outcomes. While many formulas for simple random processes that are still in use today were possibly already known in antiquity , at the latest in the late Middle Ages , the axiomatic foundation of probability theory used today only emerged at the beginning of the 20th century; The key events are, on the one hand, an exchange of letters between Blaise Pascal and Pierre de Fermat in 1654, commonly regarded as the birth of classical probability theory, and, on the other hand, the publication of Andrei Kolmogorov's textbook Basic Concepts of Probability Calculus in 1933, which describes the development of the foundations of modern probability theory completed. In between, classical probability theory had split into separate schools for centuries; these were primarily dominated by the then scientific centers of London and Paris .
Over time, stochastics has been shaped by a variety of different areas of application. Initially it was the interest of the Greeks and Romans in games of chance that drove the development of calculation models, but later suggestions came from philosophy , law and insurance , even later from physics and now primarily from financial mathematics . By way of a detour via statistics , the calculation of probability has ultimately found application in practically all quantitative sciences .
Starting position
Stochastics developed more slowly and with less determination than other mathematical disciplines such as analysis . From the beginning she had to contend with serious problems, partly due to the peculiarities of the concept of probability itself, partly to reservations on the part of other sciences such as theology , philosophy, and even mathematics itself.
Definition of probability
One way of using mathematics is to try to quantify the world. While the concretization of number concepts for sizes such as length, mass or time by a measurement - that is, the comparison with a ( normalized ) unit as the standard unit of a base size - succeeds, the quantitative detection of probabilities for a long time remained problematic. It was not until 1933 that Kolmogorow's axioms succeeded in defining a probability measure exactly, and thus also the concept of probability implied by it . However, this did not clarify what probability would be explicitly, but only worked out which structural features a probability measure must meet in order to be useful. The interpretation of the axioms remains an open question and there are still different views.
In the course of time, two schools of thought were formed which existed independently of one another without excluding one another. The Frequentismus arose during the investigation of gambling as a standardized and repeatable as many times under the same conditions, random experiments . Here, the observation showed that the relative frequency of an experiment's outcome converges with an increasing number of repetitions . According to the frequentist definition, the probability of an event corresponds exactly to this limit value - or as the French stochastic Paul Lévy put it: “Like the mass of objects, probability is a physical quantity, and frequency is a measuring instrument for this quantity, like all physical measuring instruments is afflicted with certain unpredictable measurement errors. ”As plausible as this definition is in the case of games of chance or in physics, it seems unusable for processes that cannot be repeated.
This problem does not exist if one uses the probability concept of the second school of thought, Bayesianism . Here probability is a measure of how confident you are that a certain event will occur. Formally, it does not matter whether the event is actually random or whether the outcome is simply unknown. This pragmatic approach makes it possible to dispense with philosophical preliminary considerations about the nature and existence of chance - a fact that makes this view popular, especially in statistics . A major disadvantage is that the definition of the viewer's beliefs introduces an undesirable subjectivity. In addition, in contrast to frequentism, the probability here cannot be mapped intuitively on a mathematically meaningful numerical scale. To illustrate this, thought experiments of the form “ How much would you be willing to bet on the occurrence of this event?” Must be used, which in turn inevitably leads to difficulties with the subject of risk aversion .
Although not fundamentally incompatible, these two ideologically different approaches have for a long time prevented a unified mathematical theory and a unified notation from developing.
Skepticism on the part of other sciences
Over the centuries, the calculation of probability repeatedly attracted the skepticism of other scientific disciplines. This can be traced back to two aspects or reasons:
- the terms chance and probability can only be defined and scientifically quantified with difficulty.
- Any attempt to stochastically interpret phenomena that could otherwise not or only inadequately be predicted (such as the weather , stock market prices or the outcome of a die roll ) could be seen as competition with another science.
On the part of theology and the church, for example, the attempt to use probability calculations to get closer to the “unfathomable ways of the Lord”, which one could observe every day in nature, has long been called blasphemy - the terms chance and fate are closely related. In addition, the church was bothered by the fact that in the early years the main area of application was in gambling, which it had always rejected. It seems remarkable that random processes play a role both in the old (oracle stones Urim and Thummim , Exodus 28:30) and in the new testament (in the choice of Matthias as successor to Judas by drawing lots, Acts 1, 23-26) when it it's about fathoming God's will. In the tradition of the conflict between Christianity and stochastics, there is ultimately the ongoing debate about evolution and creationism or intelligent design . The theory of evolution sees the development of living beings as the result of a randomized optimization process driven by random mutations , while creationists assume a fixed plan of creation behind it.
However, the natural scientists of the Enlightenment were also skeptical about stochastics, as they viewed them as a “ declaration of bankruptcy ” before nature. After all, all phenomena can be fully explained by deterministic laws of nature , if one only measures precisely enough and explores all laws through experiments . Thus there is no such thing as chance at all, which also excludes the existence of a serious probability calculation.
Even within the mathematician community, the idea of a probability theory was not entirely undisputed. The contradiction between stochastics as the science of uncertain events and the claim of mathematics as the teaching of true statements, irrefutable conclusions and reliable knowledge seemed too obvious. Example: Either a variable has the value five or it doesn't. In the first case, the probability of the event is 1 or 100 percent, otherwise it is 0 percent, and there seemed no room in mathematics for values in between. Even Bertrand Russell , Nobel Prize winner for literature and thought leader in the philosophy of mathematics in the early twentieth century ( Principia Mathematica , 1910), believed: “How can we speak of the laws of probability? Isn't probability the antithesis of any law? ”Only the exact axiomatic justification of stochastics in the years 1901–1933 was able to finally resolve this contradiction.
Stochastic Paradoxes
An additional obstacle in the development of probability theory was that the calculated results often run counter to human intuition . Particularly in connection with stochastic independence and conditional probability , there are many cases that lead to apparently contradicting or nonsensical results. Such phenomena are commonly referred to as stochastic paradoxes , although the term paradox is not always applicable here.
- In the prisoner's paradox , people A, B and C are sentenced to death, but one of the three is pardoned by lottery . Thus the probability for A to survive is the same . However, if the prison guard A names the name of one of the two fellow prisoners who will not be pardoned (at least one of the other two people is guaranteed to be executed) after the lottery has been drawn, then only two candidates remain for the pardon and the probability of survival for A should therefore increase . However, it is hardly conceivable that this information (after all, A knew beforehand that at least one of the others would be executed) should actually increase A's chance of a pardon, and in this case it is not so: the probability of survival is still . However, at the same time the probability of survival for the fellow prisoner not mentioned has risen to.
- The spherical paradox , also called the Bertrand paradox , was set up in a textbook by Joseph Bertrand in 1889 . It reads: if a point is randomly evenly distributed on the surface of a sphere (e.g. the point of impact of a meteorite on the earth), what is the probability that this point will come from a previously selected point (e.g. the Eiffel Tower completed in the same year ) has a distance of less than 10 angular minutes , so that the Eiffel Tower and the point of chance with the center of the earth form an angle of less than degrees? One way to calculate this probability is to divide the area of the points in question (i.e. the surface of the cap around the Eiffel Tower with a radius of 10 angular minutes) by the total surface of the sphere, which gives approximately . But Bertrand suggested a second solution: since it is irrelevant for the distance on which great circle through the Eiffel Tower the point lies and all great circles are equally probable, it is sufficient to consider such a great circle as an example. Then the probability is simple , since out of 360 degrees exactly 20 arc minutes or degrees are possible. In Bertrand's view, neither of the two answers was wrong, only the uniform distribution on the manifold of the spherical surface was not well defined .
While the prisoner's paradox can still be resolved with relatively simple stochastic aids, the second problem proves that, even at the end of the 19th century, probability theory was not yet developed enough to reproduce random phenomena on a continuum without any doubt.
But it is not only the conditional probability that plays a role in one or the other form of the paradoxes mentioned that sometimes leads to fallacies ; the concept of stochastic independence often runs counter to intuition. The following simple game is an example: an ordinary, six-sided dice is thrown twice in a row and the numbers added. The game is won if the sum of the eyes is an even number , otherwise the player loses. Now the outcome of the game (ie whether the event "game won" occurs or not) is independent of the outcome of the second throw. Although this result can easily be verified using the definition of stochastic independence, it is astonishing in that the second throw finally decides the game.
Even if these problems seem more like mathematical gimmicks today, it should not be neglected that a fully developed and consistent theory of probability is already available today. However, terms such as independence and conditional probability first had to be defined, which is difficult if the only meaningful definitions from today's point of view can lead to fallacies such as those mentioned above. This may explain why a consistent mathematical theory of probability did not develop earlier.
Calculation of probability in ancient times
An interest in chance can be traced back to the earliest human history. Archaeological finds show a striking accumulation of sheep's ankle bones and other similarly shaped bones in several places around the world . These bones, called astragali in Latin , are known to have been used as dice in the Roman Empire - for gambling for money, but also for ritual purposes to obtain information about the whims of the gods. Such and similar oracles , which use natural ( e.g. bird 's eye view ) or artificial random events, can be observed worldwide.
It is noticeable that cubes in today's usual cube shape or as tetrahedra were made early on . One of the earliest finds in present-day Iran dates back to around 3000 BC. This means that even then, attempts were made to specifically influence probabilities in order to design fair and therefore particularly interesting games. Seen in this way, the attempt to create ideal cubes - i.e. those in which all sides have the same probability - can be described as an early form of stochastic calculus. In India at least since the Vedic period before 1000 BC there were Known ritual and social games in which five-sided nut fruits were used as cubes before (limited ideal) prism cubes were developed. In the story Nala and Damayanti from the epic Mahabharata , two stochastic topics are mentioned in addition to dice games: On the one hand, the art of rapid counting, a kind of conclusion from a sample to the totality and a connection between dice games and this method of conclusion, which is unknown to us today.
Although the game of chance with ideal dice was known and widespread in the Hellenistic world and the basic mathematical knowledge would have made this possible already in the time of Euclid or Pythagoras , no traditional evidence of concrete stochastic calculations from this time has been found so far. On the one hand, this may be due to the fact that the concept of probability was not yet developed so far that it would have been possible to classify probability on a numerical scale, as is common today and understood in common parlance. But it may also have played a role that the ancient philosophy of science was strongly averse to empiricism . True knowledge cannot be gained from experiments , but only from logical reasoning. Probability, on the other hand, can only be experienced in experiments, and stochastics only enables unambiguous predictions to be made in connection with processes that are repeated infinitely often independently (e.g. in the case of the law of large numbers ), which in turn requires a frequentist approach to the concept of probability. Aristotle's statement in this context , that chance is fundamentally beyond human knowledge and thus also from science, was elevated to a dogma by later Aristotelians and for a long time prevented the emergence of a probability calculation in the West.
It is known that the Roman emperor Claudius (10 BC - 54 AD) was a friend of the game Duodecim Scripta , a predecessor of today's backgammon , and wrote a book about it. However, since this is no longer preserved today, it is unclear whether it was also a stochastic analysis of the game. It would be the earliest known treatise of its kind.
In addition to gambling, insurance also offered an early field of activity for probability assessments. Insurance contracts, especially for commercial trips at sea, can be concluded in Babylon and China at least into the second millennium BC. Trace back to BC. For example, such contracts are mentioned in the Codex Hammurapi (around 1760 BC). In the Roman Empire there was already a form of annuity in which a contractual partner received regular payments until the end of his life in exchange for a one-off fixed payment. Various forms of credit and interest can be identified even earlier ( Codex Ur-Nammu , 3rd millennium BC) and it can be assumed that such uncertain contracts are as old as the trade in goods itself.
Insurance contracts of this kind have certainly only come about after rudimentary probabilistic considerations regarding the profits and obligations arising from the contract, in which the probability of future events (such as the shipwreck of a salesman, the early death of an annuity or the default of a debtor) has been estimated. However, little evidence of this early form of risk management has survived, which is not surprising since merchants have always been careful to keep their mathematical models secret.
Middle Ages and early modern times
In the Christian society of the Middle Ages oracles and gambling, although still widespread, were publicly frowned upon, so that research on chance, at least officially, did not take place, especially since the sciences were dominated by monasteries at that time . It was not until the 13th century that another candidate for the first stochastic publication emerged. De vetula , formulated in hexameters and published anonymously , today attributed to the Chancellor of Amiens Cathedral , Richard de Fournival (1201–1260), describes games with three dice and explicitly lists the 216 possible combinations. The forbidden subject of the poem may have been the reason for the anonymous publication. Other authors such as the monk Jean Buteo (1492–1572, Logistica , around 1560) circumvented the ecclesiastical prohibition by speaking of "combination locks" instead of cubes, the keys of which had, for example, 4 beards with six settings each, the more so (6x6x6x6 =) 1296 different possibilities to be represented.
Cardanos Liber de Ludo Aleae
It took until the 16th century before the first verifiable stochastic publication came about. Gerolamo Cardano , Italian polymath and one of the most influential mathematicians of his time, laid the foundation for the theory of discrete random processes in his work Liber de Ludo Aleae (the book of the dice game), which was written from 1524 . Games with up to three dice are almost completely discussed here (as was customary at the time almost continuously in prose), but there are also philosophical thoughts on luck (Chapter XX: De fortuna in Ludo, about luck in the game ), taking risks and - shy (Chapter XXI: De timore in iactu, about the fear of the throw ), gambling addiction (Chapter IV: Utilitas ludi, & damna, benefits and harms of the game ) as well as a separate chapter on effective ways of cheating (Chapter XVII : De dolis in huiusmodi Ludis, about the ruse in games of this type ). In addition, card games are also discussed, which had become more and more popular in Europe from the 15th century, but which attracted Cardano's attention far less than Hazard , a dice game probably imported from the Orient by Crusaders .
For a long time Cardano was evidently not interested in the publication of his results, as he used an information advantage to regularly gain more than he used, and thereby partly to finance his studies. However, the notorious gambler became addicted to gambling and gambled away most of his fortune and reputation in his later life. His book was not published posthumously until 1663, when other scholars had recently become aware of the theory of probability.
The problem of division
It would be well into the 17th century before mathematicians successfully dealt with chance again, and as in many other sciences, the center had meanwhile moved from Italy to France. Blaise Pascal , one of the most influential mathematicians and religious philosophers of his time, described in a letter to his colleague Pierre de Fermat on July 29, 1654, two problems that his friend Antoine Gombaud , Chevalier de Méré, had brought up to him and which since then as De-Méré - or the problem of the dice ( French .problem des dés ) and problem of division ( problem de partis ) are known:
- The dice problem deals with a simple game of chance. The probability of throwing at least a six with a dice in four attempts is just over 50 percent. If, on the other hand, you try to get a double six with two dice - for which the probability is only one sixth of the one-dice case - and do six times as many, i.e. 24 throws, the chance of winning is just under 50 percent. After de Méré, however, the same probability should have come out as before, so that he suspected a calculation error.
- The division problem deals with a fictional game in which the player who first wins a fixed number of fair rounds (in which each player has a 50 percent chance of winning, regardless of the outcome of the previous rounds) wins a cash prize. However, the game is canceled before the decision is made due to force majeure , so that the amount should now be divided fairly depending on the current game status.
While the partners in the correspondence quickly agreed on the first problem that de Méré's "proportionality approach" (six times lower probability, i.e. six times as many attempts for equal chances of winning) was obvious but wrong and therefore there was no contradiction, the second caused greater difficulties, because here the question of justice was posed vaguely and first had to be formulated in a meaningful mathematical way. Ultimately, they came to the conclusion that the stake had to be divided according to the winning probabilities, and Pascal showed how these could be calculated using combinatorics and especially the Pascal triangle he had recently developed . The probability that a player wins exactly k out of n outstanding games is accordingly , with the binomial coefficient being taken from Pascal's triangle.
Leibniz had heard of the partition problem during his stay in Paris and had seen Pascal's estate. He was also familiar with the writings of Christiaan Huygens on the calculus of probability. In 1678 he formulated his own proposed solution to the problem of division in “De incerti aestimatione”. This work only existed as a manuscript and was not published until 1957. Leibniz came to a slightly different result than Pascal and Fermat, although he knew their solution. Leibniz had a different concept of justice than Pascal and Fermat, which today can be interpreted and expressed in a somewhat simplified way in the form of a performance principle: “Equal pay for equal performance”.
The problem of division was already known before de Méré and can now be traced back to 1380, and Cardano and his contemporaries Nicolo Tartaglia , Luca Pacioli and Giobattista Francesco Peverone had offered solutions for their part. The solutions by Cardano, Pacioli and Tartaglia differ greatly from Pascal's and Fermat's proposal, which is correct from today's perspective, because they argued with the means of a commercial profit and loss account or, like de Méré, with proportions rather than combinatorial. Peverone received almost the correct solution from today's perspective. How it came about, however, can only be researched when his work "Due breve e facili trattati" is made publicly available. Around the middle of the 16th century, Italian mathematicians lost their conviction that there was a “correct” mathematically determinable solution. Tartaglia expressed the opinion that the problem could be solved more juridically than rationally. Since Pascal and Fermat probably did not know anything about the efforts of the Italians, but later publications always built on their considerations, the correspondence of 1654 is considered by many to be the birth of stochastics.
Dutch school
While the correspondence between Pascal and Fermat was at the beginning of the development of modern stochastic calculus, it was not published until 1679, i.e. after the two died. The earliest stochastic publication in print is due to the Dutch mathematician and physicist Christiaan Huygens , who had heard of the discourse between the two French as early as 1655 while visiting Paris and then published his treatise De Ratiociniis in Ludo Aleae (On Conclusions in the Dice Game ) in Leiden in 1657 . Huygen's insight into the logic of the games and the question of their fairness goes far beyond what Cardano, Pascal and Fermat discussed. Even for asymmetrical games with different stakes or winnings, he found fair stakes with the help of an indifference principle (a game is fair if all parties were willing to swap their roles with the others) and developed one of the stochastic terms that are still central to this day: the expected value . This allowed the question of fairness to be reduced to the simple criterion “expected profit = stake”, which also solved de-Méré's problem of division.
With the Netherlands, the calculus of probability had reached one of the centers of the financial industry at that time and soon found its way into financial mathematics there. In Waardije van Lijf-renten naer Proportie van Los-renten (the value of annuities compared to amortization , 1671 ) , the council pensioner Johan de Witt , one of the most influential figures in Holland's golden age and also a hobby mathematician, used Huygens' methods to discuss state annuities, which at that time widows were offered. He used the first known stochastic mortality model and came to the conclusion that the pensions paid out were unreasonably high from the point of view of the state. Posterity probably owes the publication of his calculations to the fact that as a civil servant de Witt did not pursue any private financial interests, but had to justify his decision to the public. Rumor has it that the reduction in his pension he initiated was also a cause of a popular uprising in the following year, at the end of which de Witt was lynched .
Schism of stochastics in the 18th and 19th centuries
Huygens was the first foreigner to be accepted into the London Royal Society in 1663 for his achievements in the field of astronomy . In addition, he also introduced the calculation of probability in England , where it found fertile ground. Just a year later, John Tillotson , Archbishop of Canterbury , used Huygen's expected value in On the Wisdom of Being Religious to prove that belief in God is worthwhile. No matter how small the probability that God actually exists, the “game of God” has an infinitely high expected value due to the infinite profit in heaven. Inadvertently, Tillotson thereby drew his contemporaries' attention to a problem that stochastics would not solve satisfactorily for more than two hundred years. How do you deal with events with zero probability? His argument is only valid if one grants the existence of God a positive probability. The Pascal's Wager aimed at similar considerations.
First fundamental sentences
The calculation of probability in the 18th century was shaped by two important works, whereby for the first time a turning away from gambling towards other areas of application becomes clear. On the one hand, Ars conjectandi (The Art of Guessing) by Jakob I Bernoulli appeared in Basel in 1713 , an unfinished treatise that was published posthumously (Bernoulli had died in 1705) from his diaries. Building on Huygen's preliminary work, there are groundbreaking findings in the field of combinatorics (for example the term permutation appears here for the first time ) and a full discussion of the binomial distribution , but for the first time infinite sequences of identical random processes were also examined. For the special case of two possible outcomes, these are still known today as Bernoulli chains . The convergence of the relative frequency against the probability of an event was not assumed by Bernoulli as an axiom , but rather concluded in one sentence . It was on this basis that he formulated the earliest version of the law of large numbers , today one of the two most important theorems of stochastics. Bernoulli also failed to provide a precise definition of the probability, but he did not consider this to be necessary, since, in his opinion, there is no coincidence, only incomplete information. Someone who does not know about the course of the stars can therefore bet on a solar eclipse as well as on a coin toss. This view makes Bernoulli practically the first declared Bayesian. It is also noteworthy that Bernoulli's main interest, in addition to the aforementioned convergence statements, was to apply stochastics to case law, where it is ultimately important to assess the credibility of a statement based on incomplete information (i.e. to determine the probability of a true statement in the Bayesian sense ). This attempt to reconcile mathematical and legal inference was never seriously practiced.
The second major breakthrough during this period came from Abraham de Moivre , a Huguenot who fled to England . At the Royal Society he published The Doctrine of Chances in 1718 , a work that would have a major impact on the new English school of stochastics for the next hundred years. De Moivre's greatest achievement was certainly the formulation of a central limit theorem (next to the law of large numbers the second fundamental theorem of stochastics), now known as Moivre-Laplace's theorem, and thereby also the introduction of normal distribution . The latter, however, did not yet have the status of an independent probability distribution , but merely acted as a limit value for discrete probabilities. The probability-generating function of distributions appears here for the first time as an aid .
English statisticians and French probabilists
The work of Bernoulli and de Moivre laid the foundation for what became known in the following years as the theory of errors and later as statistics . In the natural sciences, where attempts are usually made to first detect laws through measurements, one came more and more often into situations where measurements were too inaccurate or (especially in astronomy) could not be repeated as often as desired, so that one had to go over to Understand errors as part of the model and treat them mathematically. Bernoulli had already shown in Ars Conjectandi that probability calculation is a suitable tool for this - regardless of whether one believes in the random nature of the errors or not.
The next significant step in this direction was taken by the English mathematician and pastor Thomas Bayes , whose main work An Essay towards solving a Problem in the Doctrine of Chances was published - also posthumously - in 1764. On the one hand, the conditional probability is formally introduced in it - up to now, independence was always implicitly assumed - which resulted in a special case of what is now called Bayes' theorem . In addition, Bayes was the first to demonstrate the duality of stochastics and statistics that is still valid today. While stochastics tries to infer the probability of future events on the basis of given distributions (in Bayes: forward probability ), the aim of statistics is to draw conclusions about the original distribution on the basis of observed events ( backward probability ). This paradigm laid the foundation for Bayesian statistics and heralded the dominance of the Anglo-Saxon school in the field of mathematical statistics (later represented by Francis Galton , William "Student" Gosset , Karl Pearson , R. A. Fisher or Jerzy Neyman ).
Meanwhile, the calculus of probabilities in its former form, which was still based on the foundation of Pascal and Huygens, seemed to reach its limits. In more and more areas of application it became necessary to deal with continuous distributions, i.e. those that can assume an uncountable number of values. However, this rules out that the individual values all occur with a positive probability, and events with zero probability were interpreted as impossible at that time. This apparent contradiction is that random experiments out of sheer impossible events should get together, the mathematicians could not dispel entirely conclusive until the twentieth century, even though they had their first experience with densities of distributions made, as far as the former integration theory allowed.
Meanwhile, research in the French-dominated continental school turned more to understanding the nature of chance and probability. It is therefore not surprising that the most important contributions at that time with Marie Jean Antoine Nicolas Caritat, Marquis de Condorcet ( Essai sur l'application de l'analysis à probabilité des décisions (1785), treatise on the application of the calculus of probabilities in decisions ) and Jean Baptiste le Rond d'Alembert (Articles on Probability in the Encyclopédie ) had authors who are now considered to be both philosophers and mathematicians. The main work from that period is Théorie Analytique des Probabilités (Mathematical Probability Theory, 1812 ) by Pierre-Simon Laplace , which on the one hand summarizes all the successes achieved up to that point in the field of stochastics, on the other hand it dares to attempt a new philosophy of chance. Laplace's access to the likelihood was intuitive, since he suspected behind all phenomena an equal distribution (see uniform distribution that do not use the same named Laplace Laplace distribution is to be confused). Sometimes Laplace's concept of probability is also viewed as an autonomous, third approach to frequentism and Bayesianism. In addition, he also indicated the limits of human knowledge in the field of natural science ( Laplacian demon ), with which he moved away from the philosophy of science of the Enlightenment, which had dominated the last centuries, in favor of a physics of chance.
More significant breakthroughs recorded this year Carl Friedrich Gauss and Adrien-Marie Legendre , the 1795 or 1806 independently by the least squares method developed on the basis of normally distributed errors, Siméon Denis Poisson , a student Laplace ( Poisson distribution ), and Pafnuty Chebyshev ( Chebyshev inequality , generalization of the law of large numbers), which, supported by the French mathematician Joseph Liouville and von Poisson, founded a Russian school based on the French. In addition, towards the end of the 19th century there was also a less influential German school, whose main work Principles of Probability Calculation (1886) by Johannes von Kries attempted to unite stochastics with Kant's ideas and, for this purpose, used a mathematical theory of leeway , which, however, developed according to von Kries death could not spread, although von Kries ideas should influence the later work of Ludwig Wittgenstein .
Axiomatization and basic concepts
The theory of probability had clearly reached a dead end towards the end of the 19th century, as the theory, which had been compiled in piecework for centuries, no longer met the increasingly complex demands of application. In physics, an early prototype of deterministic science, the idea of explaining phenomena through random processes at the molecular or atomic level was increasingly gaining ground.
However, three closely related events at the turn of the century led stochastics out of this dilemma to the structural framework that is now understood in the narrowest sense as probability theory. First, the development of modern set theory by Georg Cantor in the years 1895–1897, which allowed analysis to achieve a previously unknown degree of abstraction . Second, there was the list of 23 problems presented by David Hilbert at the international mathematicians' congress in Paris , the sixth of which dealt explicitly with the axiomatization of probability theory and physics and thus drew the attention of a broad spectrum of mathematicians to this problem. The third and decisive contribution was the development of the measure theory by Émile Borel in 1901, from which a little later the integration theory according to Henri Léon Lebesgue arose.
Although Borel and Lebesgue initially only wanted to consistently extend the integral calculus to spaces such as the or more general manifolds , it was soon noticed that this theory is ideally suited for a new form of probability calculation. Almost all the terms in measure theory have a direct logical interpretation in stochastics:
- The basic structure of the probability theory of measure theory is the probability space . In integration theory, it denotes the domain of definition of the functions to be integrated. Here it is the set of all elementary events, of which exactly one can occur at the same time - for example the six outcomes “1”, “2”, ..., “6” of a die roll.
- is a σ-algebra to and including subsets of , ie composed of elementary events events (such as the event that the cube is a straight number, so {2, 4, 6}). The σ-algebra (the name goes back to Felix Hausdorff ) does not have to contain all subsets of , but only those for which a reasonable probability can be defined.
- is a measure that assigns a probability to every event so that certain conditions are met. Since Borel measures were originally motivated geometrically as a generalization of surface areas , it is required, for example, that the empty set has the measure zero, that is . Translated into the language of stochastics, this means that the probability that none of the events listed in will occur is zero, i.e. it fully describes the experiment. Furthermore, it is reasonable to demand that the measure (the area) of the union of disjoint sets is equal to the sum of the individual measures (areas). Here this means that if two events can never occur simultaneously (like an even and an odd number in the same roll: the sets {1, 3, 5} and {2, 4, 6} are disjoint), the probability that one of the two occurs exactly corresponds to the sum of the individual probabilities. The same is required for countable, but not uncountable, associations. The only addition that can be made in probability theory in relation to the ordinary measure theory must, is the standardization of the entire space on one chance, so .
- Sets whose measure is zero are called zero sets, such as a straight line in the plane that has no surface. In probability theory one says of zero sets that they will almost certainly not occur. This solves the dilemma described above that random experiments can be made up of nothing but impossible events. A plane is also composed of many parallel straight lines, each of which has an area of zero. However, since there are uncountably many straight lines involved, there is no contradiction to the properties required by. This makes it possible for the first time to clearly distinguish between an event that can occur but has a probability of zero (that is a zero quantity) and one that cannot occur at all (e.g. the number seven when rolling the dice, which is not included in).
- Lebesgue expanded the theory of measurements to include so-called measurable images . These are functions with a definition set , which are in a certain way compatible with the structure of σ-algebra (for more details see under measure theory ), so that an integral can be defined for them. In stochastics, these are precisely the random variables . This replaces the mathematically unsatisfactory definition of a random variable as a "variable that assumes different values with different probability" by a solid mathematical definition.
- The (Lebesgue) integral of a function f with respect to a measure P is nothing else than the expected value E (f) of the random variable, which was already known in Huygens times .
- If one does not measure the area of a set B absolutely (that is, in relation to the whole ), but only in relation to a certain subset , this simply corresponds to the conditional probability .
- The uncorrelated nature of random variables, a weakened form of stochastic independence, corresponds exactly to the orthogonality of functions in Lebesgue space .
After the measure theory had been largely abstracted and generalized in the following years by Borel, Johann Radon ( Radon-Nikodým theorem ) and Maurice René Fréchet , the ideal framework for a new probability theory was almost a by-product. In quick succession in the first three decades of the 20th century, old stochastic sentences were translated into the new probability theory and new ones established. Problems arose, however, initially with the embedding of the conditional expectation in general probability spaces and the question of whether and how for given (infinite-dimensional) distributions corresponding probability spaces and random variables can be found that have this distribution. The young Russian mathematician Andrei Kolmogorow , an indirect descendant of the Chebyshev school and his pupil Andrei Markow ( Markow chains , theorem of Gauss-Markow ) , contributed the greatest progress in this area . Especially Kolmogorov's consistency or extension theorem , which answers the second question, was hailed as a decisive breakthrough.
Kolmogorov's textbook Basic Concepts of Probability Calculation , the first edition of which appeared in 1933, for the first time summarized the entire axiomatic probability theory developed up to that point, including Kolmogorov's extensions, and quickly became a standard work in this field. In addition to his own contributions, his greatest achievement was to bundle all promising approaches in one work and thus to provide all different stochastic schools - French, German, British, frequentists, Bayesians, probabilists and statisticians - with a unified theory. Therefore, many consider the year 1933 next to the year 1654 of the Pascal-Fermat correspondence as a possible year of birth of the probability calculation.
Modern probability theory
After establishing Kolmogorov's system of axioms, the focus in the following decades was primarily on the research of stochastic processes that can be understood as random variables with values in infinite-dimensional (function) spaces. The Brownian movement played an important role in this . Already described by Jan Ingenhousz in 1785 and later by Robert Brown when observing floating particles in liquids, this process was used in the annus mirabilis in 1905 by Albert Einstein to explain the molecular structure of water. This approach, which was very controversial at the time, finally helped stochastics to break through as an aid in physics. The American Norbert Wiener was only able to prove the existence of the Brownian movement as a stochastic process in 1923 , which is why the Brownian movement is now known among mathematicians as the Wiener process and the probability space constructed by Wiener is known as the Wiener space . The Brownian movement occupies the central position in stochastic analysis today , but most of the other processes discovered at that time were also physically motivated, such as the Ornstein-Uhlenbeck process or the Ehrenfest model .
One of the earliest studied classes of stochastic processes was the Martingale , which was originally known as roulette strategies as early as the 18th century and has now been developed by Paul Lévy ( Lévy flights , Lévy distribution ) and Joseph L. Doob (Doob-Meyer- Decomposition, Doob's inequalities) were examined in a new context. This later gave rise to the term semimartingales , which today forms the basic structure of stochastic analysis. A completely new stochastic interpretation for the σ-algebra was also introduced via the term Martingale, which Borel and Hausdorff had previously only had the rank of a technical aid. The set of all events that are known at a certain point in time (for which the question of whether they occur can already be clearly answered with yes or no at this point in time), in turn, forms a σ-algebra. Therefore, a family of temporally arranged σ-algebras, called filtration , can be used to represent the temporal information structure of a process. Such filters are now an indispensable tool in stochastic analysis.
Another class that was studied extensively early on are the Lévy processes , in which, alongside Lévy Alexandr Chintschin ( theorem of Lévy-Chintschin , laws of iterated logarithm ), the greatest successes were recorded. Chinchin had shared a doctoral supervisor with Kolmogorov , Lévy with Fréchet.
After the Second World War, financial mathematics played an increasingly important role in basic stochastic research. As early as 1900, five years before Einstein, in his dissertation Théorie de la Speculation , Louis Bachelier tried to calculate option prices on the Paris stock exchange with the help of a Brownian movement , but this caused little attention. The Japanese Itō Kiyoshi ( Lemma of Itō , Itō processes ) achieved an important breakthrough when he founded stochastic integration in the 1940s , an essential tool in modern financial mathematics, without the groundbreaking contributions such as the development of the Black-Scholes model for share prices by Fischer Black , Robert C. Merton and Myron Scholes ( Nobel Economics Prize 1973) would not have been possible. The arrival of the Brownian movement in financial mathematics showed many surprising parallels between physics and economics: The problem of evaluating European options in the models of Bachelier and Black-Scholes is equal to the problem of heat conduction in homogeneous materials.
Another mathematical aid that has found its way into stochastics via financial mathematics is the change of measure. If one always started from a fixed probability measure and then constructed stochastic processes that fulfill certain properties (such as Martingales), a suitable probability measure is now also sought for processes that have already been defined, so that the process considered under the new measure the desired Properties met. A central theorem, which establishes the connection between the existence and uniqueness of certain martingale measures and the possibility of arbitrage in stock markets, is known today as the fundamental theorem of asset pricing .
literature
- swell
- Thomas Bayes, An Essay towards solving a problem in the Doctrine of Chance . London, 1763 PDF, 920 kB
- Jakob Bernoulli , Ars Conjectandi . Basel, 1713. Translation into German by R. Haussner, Oswald's Classics of Exact Sciences , Volume 107, Leipzig 1899 ( annotated excerpt, PDF, 100 kB )
- Gerolamo Cardano, Liber de Ludo Aleae . Lyon 1663 ( PDF, 1.57 MB )
- Christiaan Huygens, De Ratiociniis in Aleae Ludo . Academia Lugduno-Batava (University of Leiden), 1657 English translation from 1714, PDF, 96 kB
- Andrei Kolmogorow, Basic Concepts of the Calculus of Probability . Springer, Berlin 1933, reprint 1974, ISBN 3-540-06110-X
- Pierre-Simon Laplace, Théorie analytique des probabilités . 4th edition. Gabay, Paris 1825, reprint 1995, ISBN 2-87647-161-2
- Representations
- Rondo Cameron, Larry Neal, A Concise Economic History of the World . Oxford University Press 2002, ISBN 978-0-19-512705-8
- Lorraine Daston, Classical Probability in the Enlightenment . Princeton University Press 1988, ISBN 978-0-691-00644-4
- Michael Heidelberger, Origins of the logical theory of probability: von Kries, Wittgenstein, Waismann . International Studies in the Philosophy of Science, Volume 15, Issue 2, July 1, 2001, ISSN 0269-8595 ( PDF, 151 kB )
- Robert Ineichen, Cube and Probability - Stochastic Thinking in Antiquity , Spektrum Verlag 1996 ISBN 3-8274-0071-6
- Ian C. Johnston, And still we evolve. A Handbook for the Early History of Modern Science . Malaspina University-College, British Columbia 1999.
- Øystein Ore , Cardano. The gambling scholar . Princeton University Press 1953.
- Glenn Shafer, Vladimir Vovk, The origins and legacy of Kolmogorov's Basic Concepts . Probability and Finance project, Working paper, 2005 ( PDF , 544 kB)
- Helmut Wirths, The Birth of Stochastics . Stochastics in School, Year 19, Issue 3, October 1999 [1]
Web links
- Electronic Journal for History of Probability and Statistics
- Materials for the History of Statistics (University of York)
- Figures from the History of Probability and Statistics (University of Southampton)
Individual evidence
- ↑ Shafer / Vovk 2006, p. 12
- ↑ Daston 1988, p. XV
- ↑ Simon Singh: Fermat's last sentence . 11th edition. Deutscher Taschenbuch Verlag, Munich 2006, p. 63 ISBN 978-3-423-33052-7
- ↑ Gabor J. Szekely: paradoxes , Verlag Harri German. 1990
- ^ Joseph Bertrand: Calcul de probabilités . Gauthier-Villars, Paris 1889
- ^ Richard J. Larsen, Morris L. Marx: An Introduction to Mathematical Statistics and its Applications . 3. Edition. Prentice-Hall, London 2001, p.3, ISBN 0-13-922303-7
- ↑ R. Ineichen, p. 15ff
- ^ R. Haller, Zur Geschichte der Stochastik, In: Didaktik der Mathematik 16, pp. 262–277.
- ^ I. Hacking, The emergence of probability. London: Cambridge Press, 1975, p. 7, ISBN 0-521-31803-3
- ↑ R. Ineichen, p. 19
- ↑ Wirths 1999, p. 10
- ↑ Wirths 1999, p. 7.
- ^ Barth, Haller: Stochastics LK. Bayer. Schulbuchverlag, 6th reprint of the 3rd edition 1985, p. 71
- ↑ Wirths 1999, p. 14 and p. 29
- ↑ Wirths 1999, p. 8.
- ↑ Wirths 1999, p. 13
- ^ Johnston 1999, Section 4, Note 5
- ↑ See for example Friedrich Fels: Comments on the concept of probability from a practice-oriented point of view . Working paper 51/2000, FH Hannover 2000, ISSN 1436-1035
- ↑ English translation in Paul Cootner: The Random Character of Stock Market Prices. MIT press, 1967, ISBN 0-262-53004-X